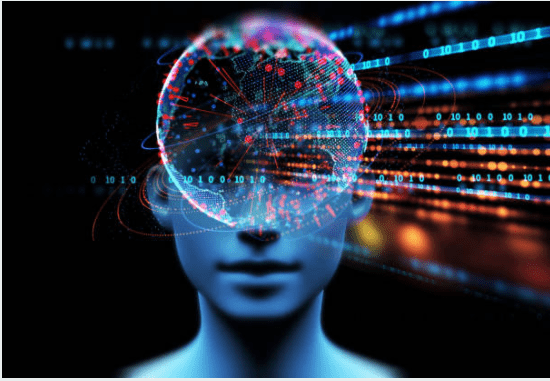
AI in real estate – Don’t Believe the Hype
Note – this article was originally published on Unissu, and is available here.
Artificial intelligence has been a technical and cultural buzzword for decades. In the past few years, AI has developed from science-fiction into something with real benefits. Despite this, it is hard to imagine a good example of what AI is, or what it can do.
We’ve all heard of machine learning, but what actually is it? The renowned computer scientist, Larry Tesler (who invented copy and paste), is commonly quoted as saying, “Artificial Intelligence is whatever hasn’t been done yet”. It is hard to provide a good example of AI because the boundaries keep changing!
Deloitte’s 2020 commercial real estate outlook (Deloitte Center for Financial Services, 2020) states that “companies should more fully embrace artificial intelligence”. Furthermore, in PropTech 2020: the future of real estate, Baum et al. (2020) suggest artificial intelligence and machine learning are some of the major technologies driving the fourth industrial revolution.
It is hard to provide a good example of AI because the boundaries keep changing!
Clearly, we should be embracing AI in all areas of business. However, AI adoption in real estate is still relatively low. The commercial real estate outlook also states, “AI is in early stages of adoption … however only four in 10 of the surveyed executives believe AI can benefit CRE development”.
I believe a lack of clarity regarding AI is hindering this adoption. This is exacerbated by headlines about scientists complaining about AI, or articles about AI and ethics. The discussions raised by these articles are relevant to the broader AI research community but are far removed from the commercial adoption of AI.
The aim of this article is to provide more clarity on AI, in the hope that a better understanding will allow the sector to:
- make more informed decisions
- accelerate the adoption of AI
- avoid being taken in by headlines and buzzwords
AI as a concept is simple. AI revolves around getting machines to demonstrate intelligence: the ability to take in information, retain it, and then apply it. There are a few fundamental approaches involved in AI research.
The individual relevance of these approaches has waxed and waned over the past few decades. The original approach to AI, in the 1940s, was an amalgamation of neuroscience and information theory, with the aim of creating machines with simple intelligence.
These machines were created with hardware-based “feedback loops” allowing for robots with basic intelligence without any computers. The idea was to replicate something like a human brain, but this idea fell out of favour by 1960.
Symbolic AI
The next approach to AI was symbolic AI, which is the easiest to understand. Symbolic AIs are based on the idea that intelligence could be boiled down to understanding physical patterns and being able to combine and manipulate these patterns. These symbols can be anything, but a common example are logical operators: words like “and”, “not”, “for each” etc.
Proponents of symbolic AI believed enough of these operators could be combined with “if” statements to create a working intelligence. This is very similar to how humans solve problems. The problem “How do I prepare for the weather?” could be solved with a series of if statements. If one outcome is true do option A, if false do option B. The if statements can even be nested (stacked) to give more flexibility. Figure 1 gives a great example of this.
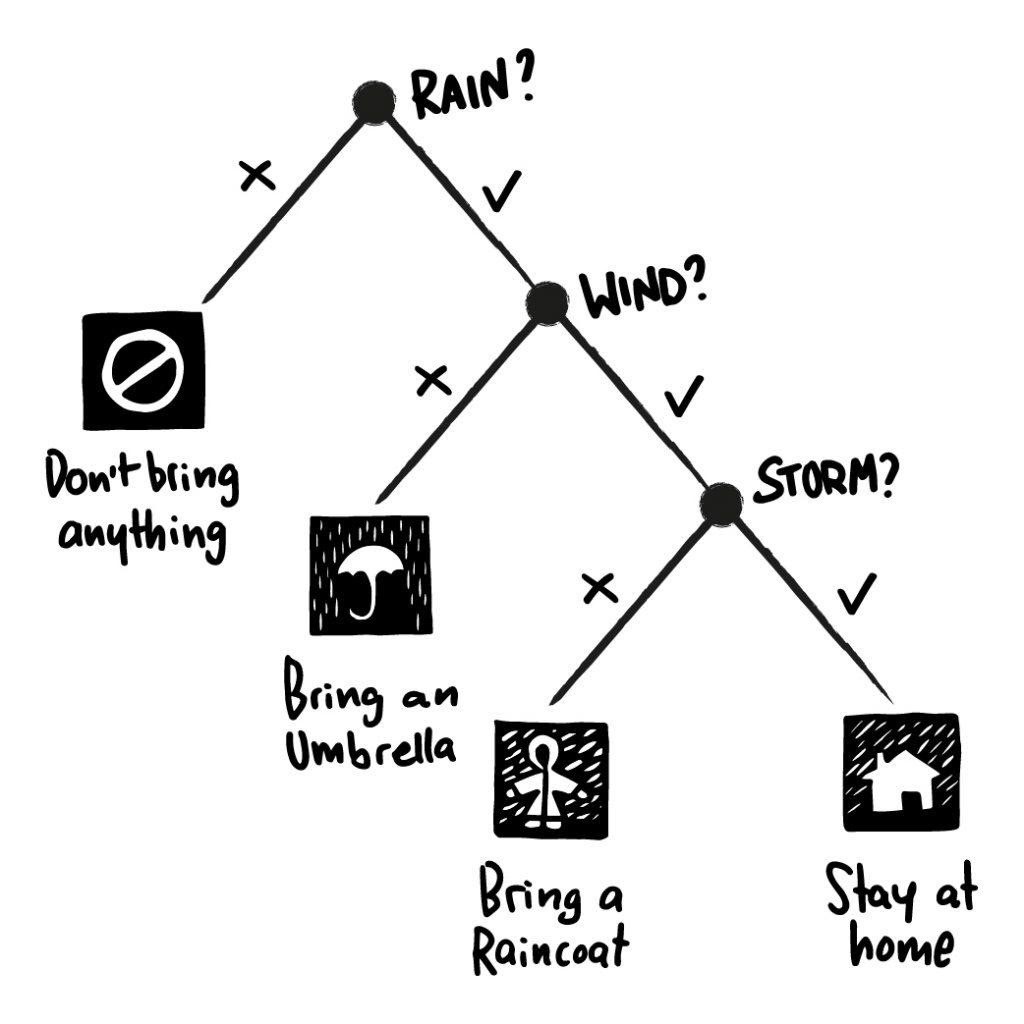
Fig. 1. An example of an If-Then decision tree (Divisio, 2019)
Fig. 1 shows the combination of ifs which lead to a decision being made. These structures are called decision trees and show how a simple series of rules can create some form of intelligence.
The issue with this is actually creating the rules. “How do I prepare for the weather?” is a trivial problem, but even that requires several rules for a computer to solve. Developing something resembling human intelligence based on these rules would be impossible. However, these human intelligences are what many people associate when they think of AI.
Popular culture is full of references to these and they form one particular area in the field of AI research – Artificial General Intelligence. AGIs are the science-fiction concept we’re all familiar with, and when people argue that AI is a risk to human existence (including Stephen Hawking, Bill Gates and Elon Musk), they are referring to this. AGIs are still decades from being done (if possible), and experts now believe these would be a combination of symbolic AI and statistical learning.
Statistical learning is now the most common approach to AI and it is also known as machine learning, a term many of us will have come across. Machine learning gives us the ability to analyse vast swathes of data and find some form of meaning from them.
Machine Learning
The key beauty in machine learning is that the algorithm is able to “fine-tune” itself after the initial analysis. The machine learns from the outputs and changes its process to make it more accurate. Sadly, a full review of machine learning and its subfields is beyond the scope of this article. Put simply, machine learning involves finding patterns in data*.
Machine learning is unlike previous fields of AI research, where the aim was to directly further the field of AI. By itself, machine learning has many commercial applications.
Machine learning is responsible for the recent developments in AI applications and adoption. This is partially philosophical – machine leaning as a field was specifically designed to address practical problems.
This is unlike previous fields of AI research, where the aim was to directly further the field of AI. By itself, machine learning has many commercial applications. Predictive analytics (the application of machine learning to commercial problems) is being used heavily in financial services (American Express and Experian are both notable adopters) and in other industries.
Machine learning has also been used to develop new technologies, which have direct benefits to real estate. We take many of these technologies for granted, and some of these include:
Optical Character Recognition (OCR)
OCR is the conversion of images into machine readable text. This has been available for some time, but earlier versions required training the software on specific fonts. Modern versions use neural networks (a specific type of machine learning) to speed up training and increase accuracy.
Contemporary OCR platforms can quickly and accurately parse text. An example of this is Xero, with their receipt scanning functionality. Pictures of receipts can be uploaded, and Xero very quickly scan the receipt and find the relevant information. This removes the need for manual data entry.
Speech recognition
Similar to OCR, but speed recognition is the ability for a machine to identify spoken words and phrases and convert them into a machine-readable format. Like OCR, machine learning has enabled the field to reach new benchmarks. In 2017, Microsoft reached a level of speech recognition which is as accurate as 4 human professional transcribers working together.
Speaker recognition
Speaker recognition is a distinct field from speech recognition. Speech recognition aims to understand content, whereas speaker recognition aims to identify the speaker. It is used as a way of authentication and verification. HSBC and Barclays have both implemented speaker recognition as a way to authenticate their customers.
Natural Language Processing (NLP)
NLP is the study of programming computers to actually understand language data. OCR and speech recognition can take images and voice to a machine-readable format. NLP is the next step and converts that machine-readable text into something that the computer can understand and make sense of. Initially, researchers believed language-processing could be done by creating grammar for computers to use.
This was unsuccessful, and now NLP is done entirely through machine learning. Machine learning algorithms are given huge bodies of text to read, and they “learn” how to understand the language from doing this. Language-processing systems trained in this way can even identify incorrectly spelled words and work around them.
There is a lot of potential for NLP in real estate. For example, it could be used in residential and commercial property management to analyse messages and emails from tenants. These could then be actioned or escalated accordingly, but with a significant efficiency boost. The combination of speech recognition and NLP have also led to two very common AI applications: chatbots and virtual assistants.
Chatbots and virtual assistants
Chatbots and virtual assitants are two related but slightly different AI applications. Both of these involve a communication platform between the user and the AI. Chatbots are very narrow and are specific to a particular company. For example, eBay have their own specific chatbot. Users can speak to this chatbot and ask it to search eBay or even buy things for them.
Any real estate company with a large customer support department could get a chatbot to automate the majority of the questions.
Chatbots are an incredible opportunity for all consumer facing elements of real estate. Any real estate company with a large customer support department could get a chatbot to automate the majority of the questions. Complex questions can be escalated to an actual employee.
Virtual assistants are similar to chatbots, as both have a communication platform between the user and the AI. These assistants are broader and more complex than chatbots and include Amazon’s Alexa and Apple’s Siri. A real estate example of this is AskPorter, who are trying to create a property management specific virtual assistant.
Computer vision
Computer vision involves AIs trying to understand images or videos. This can be used to classify images, detect things, track things and more.
This field has a lot of potential for real estate. Computer vision tools could be used to analyse marketing material for properties, and determine which images are useful and which aren’t. This could also be used to scan images for any maintenance defects, and then classify the defect based on its appearance.
Summary
There are many areas where AI can help the real estate sector. In this article, I have focused on a small selection to demonstrate some of the examples and value-adds which are possible. Hopefully a better understanding of AI and what is possible with it will empower real estate executives to fully explore this opportunity and take advantage of the countless applications of this technology.
*for more information on data analysis and how machine learning is just one facet of a comprehensive data analysis strategy – http://13.41.238.120/how-proptech-can-help-property-managers-level-up-their-data-analytics/